Integrated Glucose Control in CHO Culture via Raman Spectroscopy
Process analytical technology (PAT) and quality by design (QbD) guidelines, published by the US Food and Drug Administration (FDA) and the European Medicines Agency (EMA), emphasize that quality cannot be tested into a product but must be deployed throughout process development. These approaches are being leveraged in the biopharmaceutical industry to ensure quality is designed into processes and to achieve improvements with regard to final product quality, product yield, and process efficiency.
Read more about
Monitoring and Controlling Glucose Concentration in Cell Cultures
Monitoring critical quality attributes (CQA), such as glycosylation is important in ensuring the stability, immunogenicity, safety, and potency of biomolecules. Maintaining the glucose concentration at a steady level in the bioreactor is essential to control and optimize the process yield and quality, including glycosylation.1,2 Manual sampling and feeding of the bioreactor, however, are costly and time consuming and increase the risk of contamination each time the sterile boundary is penetrated. Implementation of Raman spectroscopy as a PAT tool for in-line monitoring is an excellent possibility to gain real-time information on glucose levels in the bioreactor which allows to react swiftly to changes. Combination of Raman technology with an automated feedback loop to enable full control of glucose levels at reduced response times for an optimized process outcome was investigated in a case study.
Case Study: Precise Maintenance of Glucose Concentration using the Raman PAT Platform
In this study, a ProCellics™ Raman Analyzer with Bio4C® PAT Raman Software (Raman PAT Platform) was used to implement a feedback control loop in a CHO cell culture process to monitor glucose concentration and enable maintenance of stable glucose levels without the need for manual intervention. The ability to monitor and maintain the desired glucose concentration led to improved process quality and supports proper glycosylation of the drug product.
Material and Methods
The feedback control loop was based on a direct Open Platform Communications United Architecture (OPC-UA) connectivity between the Raman PAT platform and the bioreactor control system.
FreeStyle™ CHO-S cells were cultivated in Gibco™ CD-CHO medium with 8 mM glutamine, 1‰ Gibco™ anti-clumping agent (all by ThermoFisher Scientific, Waltham, MA, USA) and 0.5% penicillin/ streptomycin in a 3 L glass bioreactor. The bioreactor was inoculated with cells at a density of 0.4 × 106 cells/mL in a starting volume of 2 L. Bioreactor settings are provided in Table 1. The DASware® control 5.4.1 (Eppendorf SE, Hamburg, Germany) software was used to control the bioreactor.
For the monitored batch, the culture was fed with a 15% v/v Gibco™ CHO CD EfficientFeed™ B (ThermoFisher Scientific) feed solution on day zero. Glutamine was added when the concentration dropped below 4 mM; constant glutamine feeding began on day three. For glucose feeding, a control loop was programmed based on the desired glucose concentration. The pump rate of the complex feed solution was controlled by a normal law (on DASware® control 5) based on the glucose concentration read by the ProCellics™ Raman Analyzer probe to maintain a glucose concentration of 5 g/L. Communication between Bio4C® PAT Raman Software and DASware® control 5 software was enabled by an OPC-UA connectivity and the function was defined as:
Pump rate = 2,000e (- [Glucose]2/5)
Control cultures were fed using Gibco™ CHO CD EfficientFeed™ B with 15% v/v on day 0 and 10% v/v on day 3, 6 and 9. When the glucose concentration dropped below 4 g/L, a highly concentrated glucose solution was added. Glutamine was also added when the concentration dropped below 4 mM in addition to a constant feed of glutamine starting on day 3.
Model building was used to correlate the reference values obtained by an automated off-line analyzer and the ProCellics™ Raman Analyzer . The spectra were preprocessed on the Bio4C® PAT Raman Software (SNV on the water region, Savitzky Golay derivative with 3 points (15 cm-1, polynomial order 2 and 1st derivative) and spectral selection (350–1,775 cm-1 + 2,800–3,000 cm-1)) to create a data set. The reference values were automatically linked to their corresponding spectra. The chemometric models for the monitoring were based on four standard fed-batch cultures (with a total of 103 points). A Partial Least Squares (PLS) model was developed for each monitored parameter using multivariate analytics modeling software. Models for viable cell density (VCD), glucose and lactate were created. The ProCellics™ Raman Analyzer with Bio4C® PAT Raman Software acquired and preprocessed the Raman spectra and calculated the process parameters including glucose concentration. Measurements were taken every 30 minutes and the pump rate was automatically adjusted as needed.
Comparison of results: Automated feedback loop vs. manual glucose control
As shown in Figure 1, the glucose concentration was precisely maintained at 5 g/L for 3 days by the programmed feedback loop. As a control to compare the accuracy of the Raman analyzer measurements to traditional off-line sampling methods, daily off-line samples were collected to measure glucose concentration.
Feeding was stopped when the maximum vessel volume was reached, and the process was stopped when the glucose concentration dropped below 1 g/L. A classical fed-batch process with manual glucose addition was performed as a control (Figure 1). Cell growth kinetics and the maximum cell density in the feedback-controlled run were comparable with the classical fed-batch run with less fluctuations of the glucose level in the feedback-controlled run. The feedback-controlled run resulted in a lower lactate concentration (1.8 g/L) compared to the control run (2.8 g/L). Given that high lactate levels can be toxic for cells, the lower values attained with the feedback loop strategy are advantageous in this context.

Figure 1Real-time monitoring of glucose, lactate and VCD for a fed-batch culture with a glucose feedback control loop strategy (Top) and for a fed-batch culture with a manual glucose addition (Bottom).
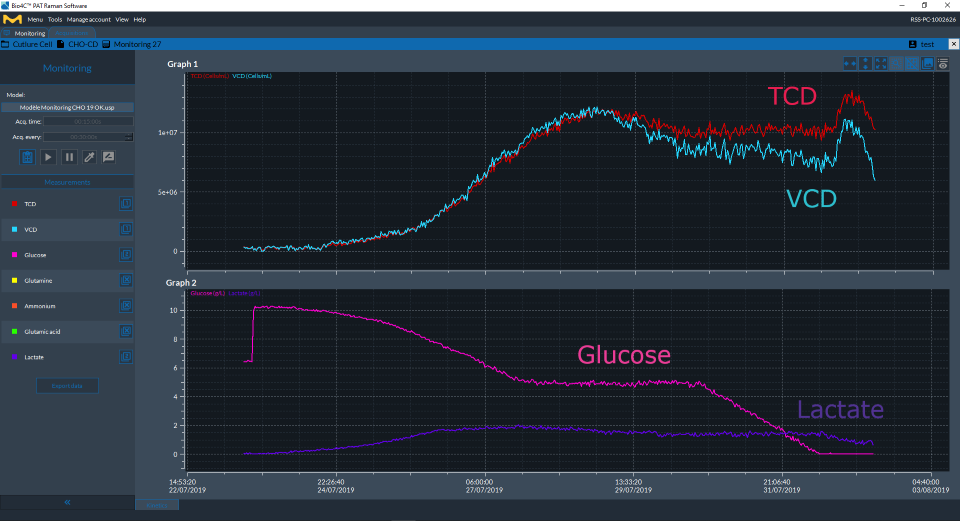
Figure 2Cell culture parameters (glucose, lactate, TCD and VCD) measured over the duration of the bioreactor culture as displayed in real time on Bio4C® PAT Raman Software.
Successful Integration of an Automated Feedback Loop for Glucose Control
By employing the feedback control loop based on measurements with the ProCellics™ Raman Analyzer the glucose concentration was steadily maintained for three days with a process performance that was similar to those of regular fed-batch cultures. The automated management of glucose concentration eliminated the need for manual sampling of the bioreactor, thereby reducing the risk of contamination. Moreover, this approach mitigated the potential for batch failures resulting from inadequate glucose levels due to gaps in manual monitoring, such as during off-hours at night or at weekends. Implementing the programmed feedback loop for glucose control enables improved and reliable process control in cell culture, empowering the user to navigate upstream biopharmaceutical manufacturing in confidence.
Request Demo
References
如要继续阅读,请登录或创建帐户。
暂无帐户?